Workbook 2
Applications of large language models
Explore the capabilities as well as potential and existing industrial applications of state-of-the-art large language models
2.1 How have large language models developed in recent years?
Over the past few years, the field of generative AI has made remarkable progress. In particular, these advances are reflected in both the significant increase in the size of Large Language Models (LLMs) and their increasing popularity (see figures below). These models have become increasingly versatile in understanding and generating different types of content, including text, images, video and source code. Recognising their significant potential, companies have begun to explore the use of LLMs in their products, services and processes across a wide range of industries, from e-commerce to healthcare, finance and more. In this way, large language models are changing how people think about AI and its ability to transform industries and society.
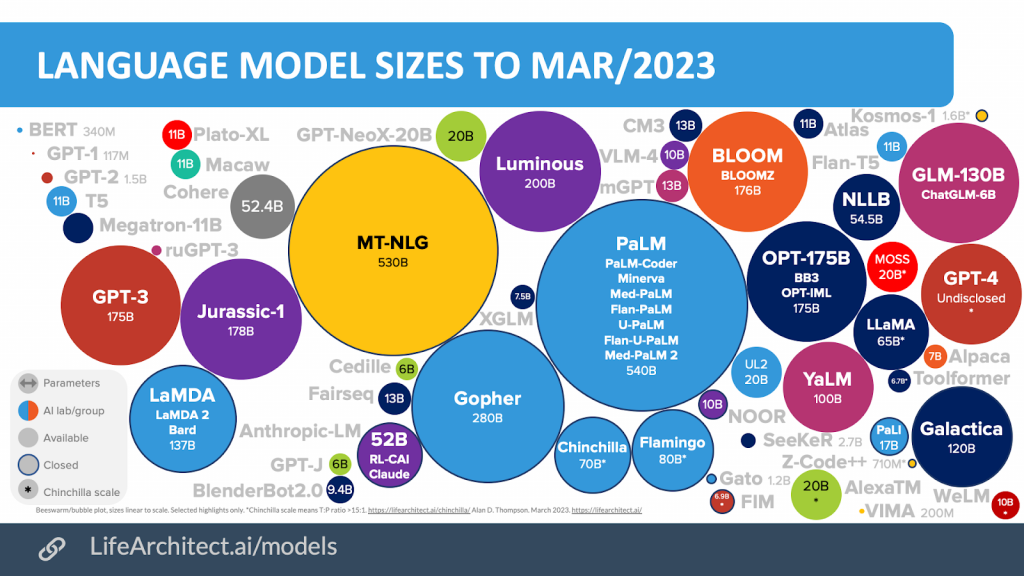
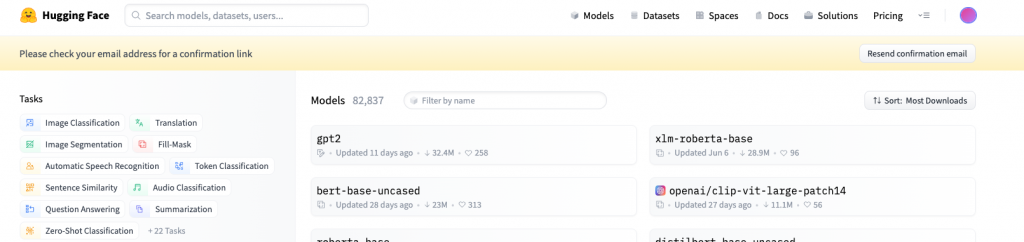
These recent developments lead to several important and impactful questions, such as: How can an organisation benefit from the use of large language models? What are some of the existing or potential use cases in products or internal processes where LLMs can have an impact? What types of results can be expected from such use cases? This chapter of the LLM Workbook aims to answer these questions by looking first at what large language models can do for industry, and then at existing or potential real-world applications of LLMs in specific industry sectors.
2.2 What can large language models do for industry?
Before exploring the use of large language models in real-world industrial applications, it’s important to have an understanding of several basic tasks that LLMs can perform in these use cases. These tasks, explained in turn below, are critical to the success of most industrial applications of LLMs. All the examples mentioned in this section, from a variety of start-ups and established companies, are publicly available.
-
Text Generation
Text generation, also known as natural language generation, is the process of using input data, such as a sequence of words or phrases, to output text that is human-readable and coherent. It forms the basis of question-and-answer systems that can generate responses or answers to user input. Text generation has become one of the most prominent tasks in natural language processing, largely due to the popularity of ChatGPT, a large language model developed by OpenAI. Since its public release in November 2022, ChatGPT has demonstrated remarkable abilities to generate coherent and human-like text from a variety of inputs, attracting significant public and media attention. This has raised awareness of the potential of text generation in many industries, from manufacturing to software, where the generation of content such as articles, news, marketing slogans, social posts, minutes, customer feedback or product descriptions is an integral part of everyday work.
With tools such as Copy.AI and Smart Copy, businesses can use the latest advances in natural language processing to automate the creation of virtually any type of content, including digital advertising copy, social media content, website copy, e-commerce copy, blog content and sales copy.
-
Image Generation
Foundation models are now also capable of generating artificial images from pre-selected examples of images or text descriptions. Known as image synthesis, this has become an important application of generative AI models. In particular, the latest transformer-based models show significant improvements in generating photorealistic images with a wide range of content, style and variation based on text-based prompts.
Examples of such models include DALL·E 2 from OpenAI and Stable Diffusion from stability.ai. DALL·E 2 is a 12-billion parameter version of GPT-3 that is trained to generate images from text descriptions, using a dataset of text-image pairs. Microsoft is integrating DALL·E 2 into its range of cloud AI services and software products, including the Microsoft Teams platform. Stable Diffusion, which was primarily developed in Germany, is a text-to-image diffusion model that has similar capabilities to DALL·E 2.
Luminous, a family of large language models developed by Aleph Alpha, takes a different approach: Instead of generating images from text, its basic and advanced versions can read, explain and generate text based on existing images. This includes contextual Q&A, image captioning, and image analysis and summarisation. These features can be tested in the Aleph Alpha Playground.
Significant advances in LLMs for image generation have led to a shift in the way AI-based content generation is viewed. Beyond pure text generation, various AI models are now focusing on multimodal generation of video, audio and even music.
-
Code Generation
Code generation is the process of translating natural language into code or translating code between different programming languages. As large language models continue to evolve, code generation has become popular in the developer community. This technology allows developers to write code with minimal human input, making it a valuable tool for building applications more efficiently.
By automatically generating code from natural language or other input, code generation technology can be used to enhance low-code/no-code apps, which have become increasingly popular in recent years. These tools allow developers to build applications without writing code by hand. Instead, they can use drag-and-drop interfaces to create workflows, forms and other features.
While it is still unclear whether AI will replace the skills of software developers or require the development of new skills, natural language code generation shows promise as a tool that can help developers work faster and more efficiently. For example, CodeX by OpenAI can generate code from natural language input, making it easier for those who aren’t programming language experts to write software. Another example is GitHub Copilot, an AI assistant that helps developers by using machine learning algorithms to suggest snippets of code.
-
Machine Translation
Machine translation has a long history, starting with rule-based solutions developed by IBM in 1954. Today, neural machine translation (NMT) is the most common approach, using deep learning to outperform statistical machine translation (SMT). German machine translation specialist DeepL has become one of the most popular machine translation services globally, with over a billion users. The popularity of machine translation continues to grow due to the ease with which it can be integrated into a wide range of end-user products,
Integrated into NMT solutions, (multilingual) LLMs improve the quality of translation including in so-called low-resource languages, i.e. languages with only a small amount of digitally available corpora data (translation pairs) for training NMT models.
Technology companies are investing heavily in research into different large language model architectures and methods optimised for machine translation. Amazon, for example, released the Alexa Teacher Model, which outperforms previous machine translation approaches and improves key services such as AWS Translate and Alexa. Alongside commercial options, open source projects like BLOOM produce coherent translations in 46 languages and 13 programming languages.
-
Text Summarisation
Text summarisation, the process of reducing large amounts of text while retaining the essence of the original, is widely used in applications that summarise news articles or meeting notes, produce reports and briefings, and even generate mind maps from text. As the amount of information available increases exponentially in the digital age, there is a growing need to quickly sift through large amounts of text to identify key points. Large language models have emerged as the most effective choice for text summarisation tasks due to their superior language understanding capabilities for long and complex texts.
The aforementioned Luminous family of LLMs from Aleph Alpha, available for testing on the Aleph Alpha Playground, is trained in the five most commonly spoken European languages (English, German, French, Italian and Spanish) and can summarise texts and documents in these languages.
-
Speech Recognition
Speech recognition, also known as automatic speech recognition or speech-to-text, is the process of converting spoken language into text. This technology enables hands-free interaction with popular virtual assistants such as Siri, Alexa and Google Assistant, and is now ubiquitous in smartphones, smart speakers, cars and even robots. But speech recognition isn’t just limited to virtual assistants – it’s also widely used in call centres and dictation-related applications, such as supporting doctors in the healthcare sector or in manufacturing.
Although speech recognition technology has made great strides in recent years, it has yet to achieve human-level performance. OpenAI has recently released a new automatic speech recognition (ASR) model, Whisper, that has the potential to change this. Whisper, which can be tried out on the Whisper Playground, demonstrates near-human accuracy and robustness, outperforming previous supervised and unsupervised models.
2.3 How are large language models used in industry?
Deploying large language models in practical, real-world industrial contexts can be challenging. Each industry has its own unique requirements, processes, vocabularies and jargon that need to be taken into account. It can also be challenging to find the right applications for large language models and to ensure that they perform well and produce robust results in these specific contexts. In this section, we look at real-world examples where large language models have already been successfully implemented with significant impact, as well as potential use cases where large language models could be useful. We cover a range of industries including healthcare, finance, manufacturing and more. Click on the interactive landscape below to navigate through the different industries and find examples relevant to your interests.